Keep Calm and Feed the Model: The Rise of Data Licensing in AI
AI needs Data. Model Developers either crawl the web or engage in licensing deals to acquire this for improved training or context enrichment. We are seeing a new model for content and data distribution emerge – distribution to AI models, applications and agents. As AI models and applications become more widely used, the economics of distribution and licensing will change. This article explores three licensing and distribution frameworks and then explains their relevance in the broader landscape of AI development using the concept of tail end economics proposed by Chris Anderson in 2001, as a supplementary theory.
Licensing, Attribution, and Tooling Frameworks
Although new, data licensing for AI has rapidly evolved from direct licensing to referral based/ad supported models. Direct licensing is straight forward; foundational model companies pay a lump sum to license the content of premium publishers to train their models. OpenAI has led the pack with the first major direct licensing deal occurring in 2021 with Shutterstock. The strategic partnership was used to train DALL-E and since expanded to a six-year agreement in 2023. These licensing agreements have evolved considerably; OpenAI’s ‘Preferred Publisher Programme’ (PPP) promises publishers in the programme enhanced discovery in the generated responses of ChatGPT, along with cash and access to generative AI tools, in exchange for training on a publisher's content.
Enhanced discovery means a new source of traffic acquisition for publishers. Attribution is how content platforms acquire referral traffic – when publishers say they want attribution what they are really saying is, ‘we want referral traffic for our content’.
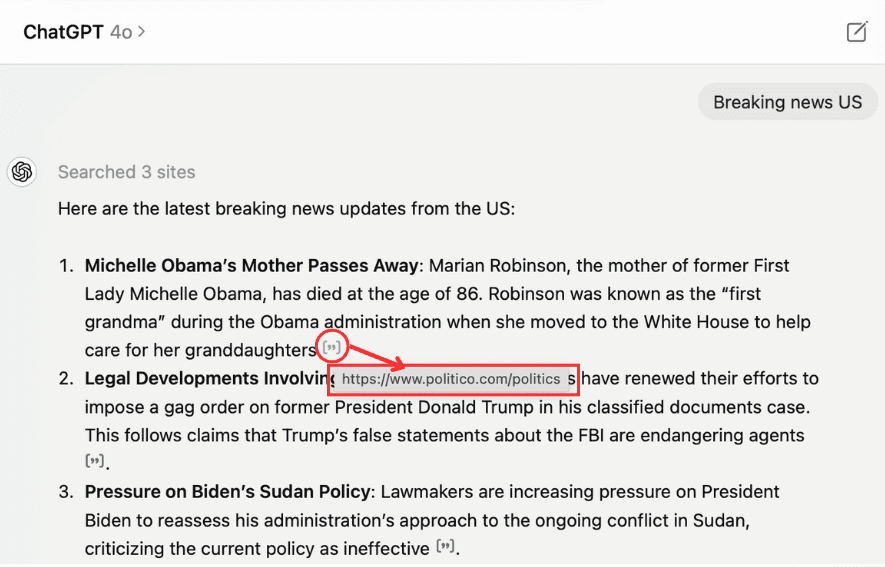
Figure 1 – A screenshot of ChatGPT on 06/24 directly referring users to Politico, a member of the PPP, through an referral link.
NewsCorp, Vox Media, the Atlantic, Politico and more signed on to the PPP. This licensing and partnership framework is unlikely to scale in the long term for a few reasons. First, this approach can only be taken on by start-ups and companies with the deepest pockets (OpenAI paid $250 Million to NewsCorp). This effectively pushes out AI developers with leaner budgets and spending capability away from responsible data licensing. Second, model developers only want to license data from premium sources indicating lower appetite for content from smaller, tail end publishers and rights holders. Additionally, data licensing for model pre training and fine-tuning is not repetitive. Once a model is trained and then fine-tuned the training data does not serve a greater purpose beyond that.
Third, for the reasons mentioned, a push towards attribution and revenue share in an inference-based system will likely eclipse both direct data licensing and data partnerships because models will already have all the data that can be acquired from our current sources and practices – we at Valyu recognise this. As frontier data is explored more and more, different licensing and cost structures could emerge.
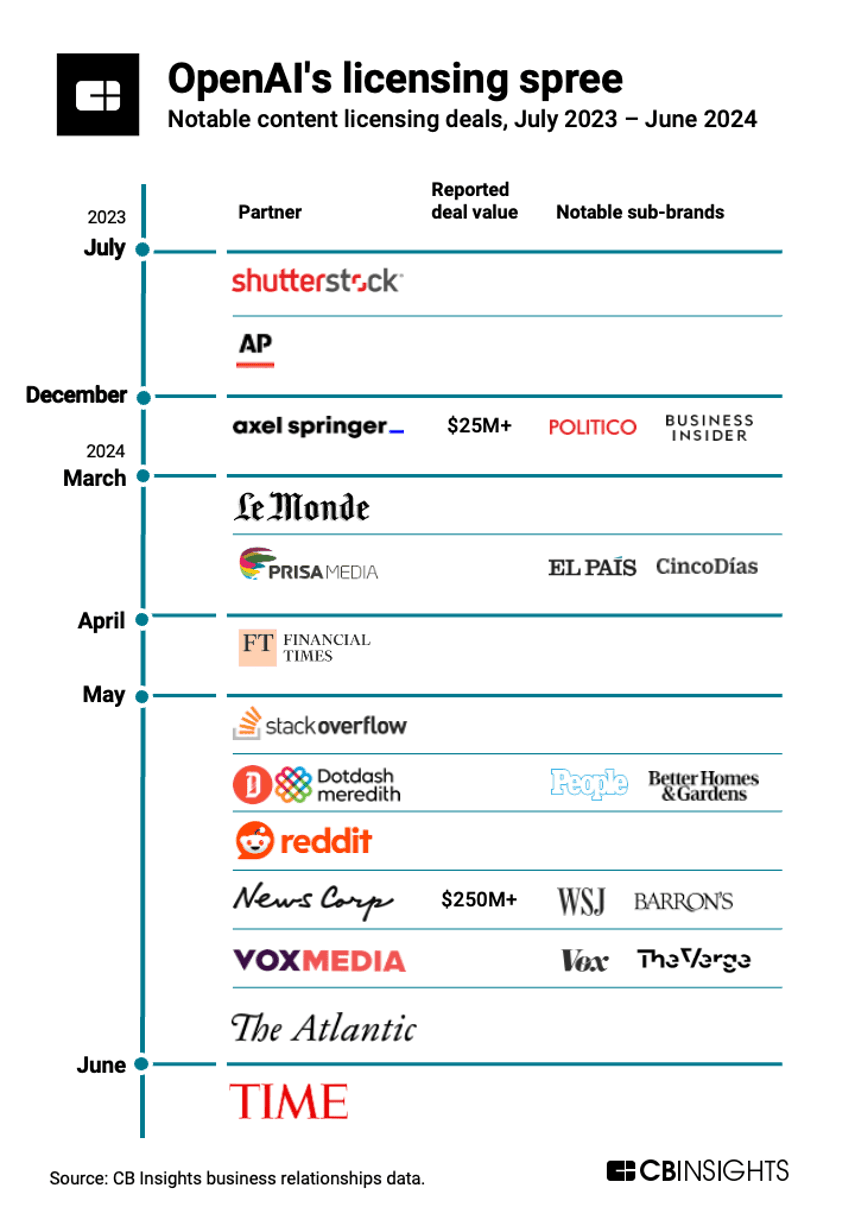
Figure 2: AI content licensing deals. Source: CB Insights
Revenue Share and Attribution is starting to be seen as the mechanism for content/data monetisation for AI. Perplexity (via ScalePost), and ProRata are taking their own respective approaches. Perplexity’s Publisher Programme has Time, Der Spiegel, Fortune, Entrepreneur, The Texas Tribune and WordPress.com already signed up. This approach to attribution is more sophisticated – It is ad supported and brands can pay to ask specific related follow-up questions in Perplexity’s answer engine interface. When Perplexity earns revenue from an interaction where a publisher’s content is referenced, that publisher also earns a share. Perplexity’s Programme began in August alongside its intense growth strategies for Linkedin and UberOne users. Similarly, ProRata has claimed to have developed a Generative AI attribution technology that is able to attribute every contributor to a generative output while maintaining factual integrity. The product is going to be released this fall. The success of these models will rely on scale to produce revenue and whether that will be reached and if the attribution frameworks are effective is yet to be seen.
Tooling is another channel that shows long-term promise to be adopted by the middle market and tail end of publishers. Cloudflare’s tooling suite enables any website hosted on Cloudflare to control which bots can scrape their website and for what purpose. While the tooling is promising in enabling smaller rights holders some level of control, particularly those that are not considered ‘premium’ content by large foundational models or possess the ability to negotiate directly with AI companies directly. But the open question remains as to whether these tools will provide value in the long run. As mentioned earlier, AI companies are unlikely to license data unless it is of high value regardless of how rights holders feel about the value of their content. Additionally, the contribution of a single piece of work into an entire training dataset is so minimal and, in some cases, insignificant, that the revenue share gained from training would be in the region of 0.0001 of a cent for a single piece of art or piece of content. This signals the unit economics of data breaks down for smaller publishers and rights holders.
If models of licensing, revenue share and attribution, or tooling are successful, this will open the availability of data for smaller developers that do not have the bargaining power or knowledge to go directly to publishers to license their data correctly. This would make licensed data for training, fine tuning, and context enrichment accessible to more developers and increase the pace innovation.
The Long Tail of the AI Marketplace
To embed the licensing and attribution channels discussed into the broader AI development landscape, I am going to introduce some theory I learnt from my flight to San Francisco from a book called “The Long Tail” by Chris Anderson, WIRED magazine’s editor and chief from 2001 – 2012. Anderson describes a distribution curve where a small number of popular products (the "head") account for a large portion of sales, followed by a "long tail" of many niche products with lower individual sales volumes.
As a business strategy, being able to supply a large number of niche products or services can collectively account for a significant portion of sales and market share, often rivalling and potentially exceeding the share of a few best-selling items. Amazon, Spotify, and Netflix are all hyper-successful examples of longtail business strategies. By being able to supply products, and content to the most niche, far reaching tastes and preference of their consumers, they are able to collect on the long tail end of their respective marketplaces. This rest of this article is going to dive into Anderson’s theory of the long tail and apply it to the current generation of AI development and explain how the evolution of licensing and attribution channels are slowly removing the current bottleneck around content and pushing models and application from the Short Head.
The concept of the long tail is counter intuitive to conventional business logic that follows the 80/20 rule (the Pareto Principle); 80% of results come from 20% of efforts, 80% of revenue comes from 20% of customers, 80% of profits comes from 20% of products or services. Anderson describes these as the “Biggest Hits” or the “Short Head”; the products that have the most generalised popularity or generate the largest aggregate return for a single product. In an underdeveloped or narrow market place most market participants or products are aiming to capture this shorthead. Useful examples are the music and publishing industries prior to the age of the internet. Record shops and Bookstores would receive 80% of their profits from top 20% of the bestselling albums and books respectively.
So where does that leave the last 80% of albums and books? Nowhere, because it didn’t make sense to appeal to the smallest market audiences, the tail end, due to the modest returns and the costs of distributing these products.
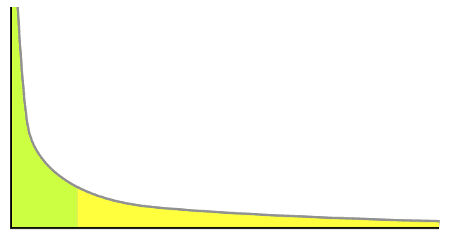
Figure 3. The Long Tail: Where green is the "Short Head" and yellow is the "Long Tail."
Traditional expectation assumed that demand for less popular products would reach zero the further to the right a product travels along the distribution curve. However, an interesting phenomenon emerged with the internet and its ability to connect supply with demand through discoverability of products.
Amazon did not hold any physical inventory, but through partnerships with major book sellers, it was able to advertise on its website a vast catalogue of books for its customers on virtual shelf space that traditional bookstores could not and would not have supplied. This enabled Amazon to fulfil requests for even the most niche book titles requested by ordering from publishers as they were requested, reducing their need for physical inventory. This was so successful that fulfilling these niche orders became a significant part of Amazon’s business model. This idea of fulfilling niche demand underpins the “Long Tail” theory, which argues that with sufficient variety and availability, even infrequent demand for niche items accumulates to form a significant market.
The Three Forces of the Long Tail
In his book Anderson Identifies three forces that are critical for the development of a long tail market. The Democratisation of Production, The Democratisation of Distribution, and being able to Connect Supply and Demand.
The Democratisation of Production refers to the process of technological advancements marking tools for creating content (such as music, film, writing, and other forms of media) affordable and accessible to the public. This allows everyday individuals, not just professionals, to produce high quality work, transforming them from consumers into creators and contributing to a shift in cultural participation and industry dynamics.
The Democratisation of Distribution refers to the way the internet and digital platforms have made it easy and affordable for individuals and small producers to distribute their content, products, or services directly to a global audience. This shift eliminates traditional barriers like expensive retail space or media gatekeepers, allowing anyone to reach a wide market through platforms like Amazon, YouTube, or social media, transforming how goods and content are shared and consumed.
Connecting Supply and Demand refers to the way digital platforms, search engines, and recommendation algorithms enable consumers to find niche products and content more easily. By removing traditional barriers such as limited shelf space and geographical constraints, online platforms like Amazon, Netflix, and Google connect consumers with a vast range of products that match their specific interests, even if those products cater to very small markets. This enhances market efficiency by allowing supply and demand to meet in ways that were previously impossible.
Where are AI Models and Applications on the Distribution Curve?
Observing the current amount of market participants developing foundational models, what use case’s they are building for, and who the end users are illustrates that the industry is still mainly in the “Short Head”. There are less than a dozen models that are commonly used and they are produced by OpenAI, Anthropic, Google, Cohere, Meta, IBM, and Nvidia. Each of these companies is building to be the “Biggest Hit” with the goal of either growth in user adoption and retention or revenue turnover.
The use case’s that foundational model companies are building for have the broadest appeal across enterprise and consumer adoption and are narrowly focused on general assistance and automation with the goal of increasing productivity and efficiency when used correctly. This is reflected in their user growth, ChatGPT currently has over 200 million monthly active users. As of March 2024, Mistrals 7B and 8x7B models have a combined 3 million downloads. Following this there are the biggest hits in individual domains; Eleven Labs in Audio generation, Runway and Black Forest labs in Video Generation to name a few. As of now, the current shorthead of AI development shows us that the force of distribution is not a problem. The internet and digital infrastructure development has maintained reasonable pace with broader innovation across telecommunications and bandwidth.
The same innovations are applicable for the distribution of AI applications and will continue to be useful for the foreseeable future. Connecting supply and demand is not quite an issue just yet because there isn’t an overabundance of AI applications and tooling that are targeted for the average non-technical consumer, they will simply use what is available. However, the tools for production have yet to democratise and there is going to be some growing pains specifically regarding data and its underlying economic model.
Data Licensing, Revenue, and Attribution Unlocking the Tail End
The question then remains is which of the previously mentioned frameworks is most likely to succeed and how does this support a developing tail end marketplace for AI applications. Using the example of Cloudflare the tooling for publishers looks to be the most promising because it addresses all three forces of the tail end specifically for data; Production, Distribution, and Connecting Supply and Demand.
Data as a tool for production is democratised by streamlining the scrapping terms directly with publishers of all sizes (larger or premium publishers are likely to still negotiate directly with developers). Distribution is democratised because data is directly crawled from the publisher to the bot belonging to the AI developer. Supply and demand are being connected, in the case of the Cloudflare example, through a centralised marketplace. Per Anderson’s theory, all three of these dimension’s would unlock the tail end of training data and by extension unlock the tail end of AI applications.
To repeat a previous, argument against the attribution and royalty approach is that an individual’s contribution to a training dataset or a generated output is so small that the royalties received would be minuscule (think 0.001s of a cent). This would not lend itself to being an effective stream of income unless entities were going to produce content at scale. Furthermore, model training isn’t really repeated on the same dataset. Once it’s consumed, it’s consumed. This makes reoccurring revenue from training or fine tuning short-lived and will bring inference into the spotlight.
Anderson’s theory of the long tail is a useful tool when observing the current AI development landscape and understanding what will need to happen to see a long tail of AI applications develop. The licensing and attribution channels discussed in this article are novel and will need to be iterated again and again, but the market is slowly working out the unit economics. As data becomes democratised as a force of production, seeing all the different use cases and applications emerge to serve a growing array of niches and interests is exciting.
—-
Cover Image by Google DeepMind from Pexels.